A look at the harmful impacts of algorithmic bias and what we need to do in order to prevent it.
If you’ve ever applied to an online job posting, you may recall uploading your resume electronically.
The company you were applying to likely got hundreds of other resumes for that particular job posting. Sifting through these applications would require a hefty amount of time and resources — if they were being evaluated by a human. Most companies, however, use Applicant Tracking Systems, or ATS, to filter out resumes that do not contain specified keywords or skills. This allows companies to easily and quickly strain a pool of applicants to find those most qualified for the job. Many have revered ATS for getting rid of — or at least reducing — bias in the hiring process.
However, the algorithms responsible for these decisions may be biased against marginalized populations. ATS can filter out otherwise qualified candidates because of their race, sex, socioeconomic status, etc., as proven possible when Amazon’s AI hiring tool discriminated against women.
This begs the question: why do algorithms show bias? And, more importantly, how can we prevent these biases?
In Shalini Kantayya’s 2020 documentary Coded Bias, MIT Media Lab researcher Joy Buolamwini speaks of her startling experience using facial recognition technology in which her face was left unrecognized by the computer’s algorithm, but was recognizable only when she wore a white face mask. This prompted her to look into how and why the algorithms used for her computer were more acute with reading a white mask as opposed to an actual human face. She found that the majority of the data that had been used to refine the algorithm used White, male faces. As a result, the algorithm could recognize lighter men with the most accuracy, followed by lighter women, darker men, and finally, darker women.
This is an example of algorithmic bias.
Algorithmic bias is a term used to describe “systematic and repeatable errors in a computer system that create unfair outcomes, such as privileging one arbitrary group of users over others.“
Algorithmic bias can be extremely harmful for a variety of reasons. The world continually adapts algorithms to make crucial decisions. Algorithms exist to help businesses decide whether or not to offer people loans, evaluate job candidates for the next stage of the hiring process, and even in assessing sentence lengths for people who have been convicted of crimes.
With the use of such algorithms comes an assumed impartiality, because these tasks are being carried out by computers. About “40% [of Americans] feel it is possible for computer programs to make decisions that are free from human bias.” We know that computers aren’t scheming against the public, nor do they have racial or socioeconomic biases — they’re just computers. However, algorithmic bias occurs when the datasets used to train such algorithms leave certain groups within a population unaccounted for.
For example, Facebook users can have their posts flagged and taken down if they violate Facebook’s community guidelines. The social media website trains its sensors to remove content that is potentially harmful to protected categories, which are defined by “race, sex, gender identity, religious affiliation, national origin, ethnicity, sexual orientation and serious disability/disease.”
However, more leniency is leveraged to users who write about subsets of these protected categories. Content against White men would be flagged, because being White and being a man both fall into protected categories. Writing harmful content about Black children or women drivers, however, would be allowed, because age and driving are not protected categories, so these populations are considered subsets. As a result of algorithms like these, a U.S. Congressman, in regards to “radicalized Islamic suspect[s],” was able to post “[h]unt them, identify them, and kill them. Kill them all. For the sake of all that is good and righteous. Kill them all.” This is because radicalized Muslims (or “Islamic suspects”) are considered a subset of Muslims.
How can we prevent algorithmic biases?
Diversity within data science professions has been proven to effectively reduce algorithmic bias. Algorithms that are utilized to make weighty decisions for the entire population should be developed by people who realistically represent said population.
A study at Columbia University divided 400 engineers into groups “in which certain engineers were given data featuring “realistic” (i.e., biased) sample selection problems while others received data featuring no sample selection problems.” Researchers found that “prediction errors were correlated within demographic groups, particularly by gender and ethnicity. Two male programmers’ algorithmic prediction errors were more likely to be correlated with each other.” This shows that having diverse groups of engineers is central to reducing algorithmic bias.
As of 2017, women held only 26% of data jobs in the U.S., according to Forbes. This can be attributed to a lack of both mentorship for women in the technology industry and STEM education for young girls. Data for the 2013-2014 school year showed that higher level STEM courses were not offered in high schools with larger Black and Latinx student populations, as depicted in the diagram below.
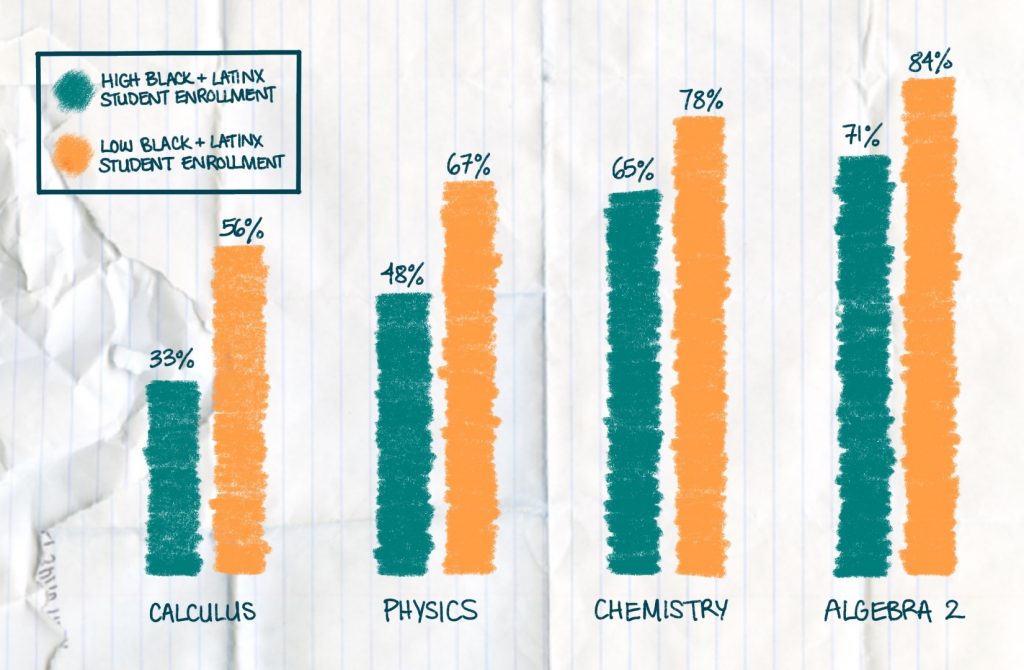
Educational disparities have kept people marginalized for years.
Skillspire’s mission is to introduce minority populations and their talents to the technology industry. We believe that diverse perspectives are crucial to the tech sector, and are clearly vital to preventing and undoing the harmful effects of algorithmic biases.
Interested in entering the tech industry? Check out our courses here!
Are you a company looking to diversify your team? Learn about our internships, apprenticeships, and Skillspire Sponsorship Program (SSP) here!